Autonomous driving (AD) and advanced driver assistance systems (ADAS) rely on the precise sensing of the vehicle’s surrounding environment to safely navigate. TERAKI has released the latest radar detection software that accurately identifies static and moving objects with increased accuracy and less computational power. The real traffic solution runs on ASIL-D compliant AURIX™ TC4x microcontrollers from Infineon Technologies AG.
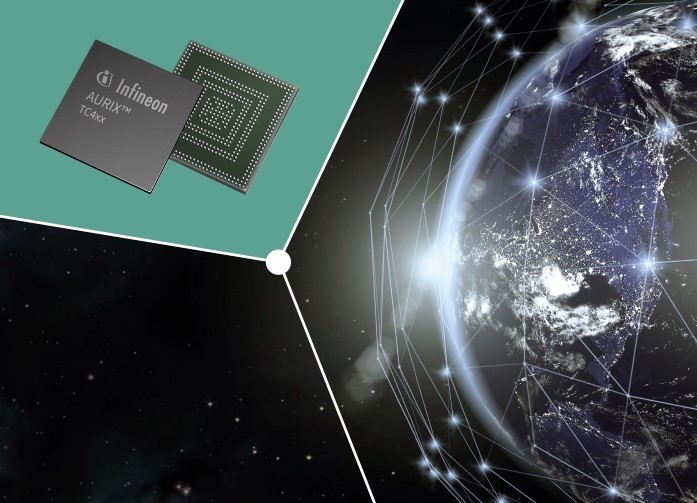
As radar turns into the industry standard for cost-effective signal processing, overcoming the limitations of this sensor technology becomes a priority. For example, interference can severely lower radar detection performance, leading to invalid detections in difficult multi-target situations, which also carries high processing requirements. Additionally, the precision required for reliable radar classifications involves more data points per frame and sub-1-degree angular resolution, if static, and moving objects to be correctly detected and classified.
TERAKI’s machine learning (ML) approach intends to solve this challenge by working with raw data and reducing noise, while acting as a cognitive function to dissect information from the radar, identify targets in a noisy environment, along with clusters and other interference, and decreasing the processing capacity at the edge. TERAKI’s ML-detection delivers more points per object, leading to less false positives and thus, increased safety; particularly when compared to other radar processing techniques, such as CFAR.
Ported with Infineon’s AURIX TC4x, TERAKI’s ML-based algorithm reduces radar signals after the first Fast Fourier Transformation (FFT), achieving up to 25 times lower error rates of missing objects at the same RAM/fps. Compared to CFAR, classification is up to 20 percent higher in precision, and valid detections increase to 15 percent more. With this release, TERAKI is improving chipset architecture of edge devices, ensuring real-time processing performance on AURIX TC4x, which alleviates the computing requirements by consuming 4- or 5-bit bitrates instead of 8- or 32-bits without compromising the F1-scores. This leads to up to 2 times less memory required.